top of page
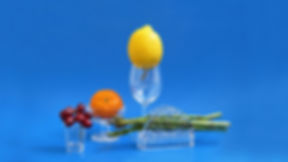
토검사
【토검사】토토사이트,안전놀이터,메이저놀이터,메이저사이트,먹튀검증,메이저토토,메이저토토사이트,토토,사설토토,스포츠토토,안전토토,안전토토사이트
토토사이트, 토토사이트 순위, 토토사이트 모음, 토토사이트 추천, 안전토토사이트, 사설 토토사이트, 불법 토토사이트, 합법 토토사이트, 토토사이트 꽁머니, 메이저놀이터, 메이저놀이터 순위, 메이저놀이터 목록, 메이저놀이터 검증, 메이저놀이터 추천, 메이저놀이터 순위 디시, 메이저사이트, 메이저사이트 순위, 메이저사이트 추천, 메이저사이트 먹튀, 메이저사이트 순위 디시, 토토 메이저사이트, 메이저토토, 메이저 토토사이트순위, 메이저토토사이트, 안전토토, 안전토토사이트, 안전놀이터, 안전놀이터 순위, 안전놀이터 모음, 스포츠토토, 사설토토, 스포츠토토사이트, 스포츠토토 온라인, 스포츠토토 사이트 추천, 먹튀검증, 먹튀검증업체 순위, 먹튀검증커뮤니티, 검증사이트, 안전 검증사이트, 검증놀이터, 놀이터토토, 놀이터 토토 도메인, 놀이터 토토사이트, 토토추천, 사설토토 추천, 사설토토사이트, 사설 토토 순위, 메이저 사설 토토, 토토추천사이트, 승인전화없는 토토사이트, 오래된 토토사이트, toto사이트, major사이트, major놀이터, 안전놀이터 추천
bottom of page